|
Detecting an abandoned object in crowded scenes of surveillance videos becomes more complex task due to occlusions, lighting changes, and other factors. In this paper, a new framework to detect abandoned object using dual background model subtraction is presented. In our system, the adaptive background model is generated based on statistical information of pixel intensity that robust against lighting condition. Foreground analysis using geometrical properties is then applied in order to filter out false region. Human and vehicle detection are then integrated to verify the region as static object, human or vehicle. The robustness and efficiency of the proposed method are tested on several public databases such as i-LIDS and PETS2006 datasets. These are also tested using our own dataset, ISLab dataset. The test and evaluation result show that our method is efficient and robust to detect abandoned object in crowded scenes.
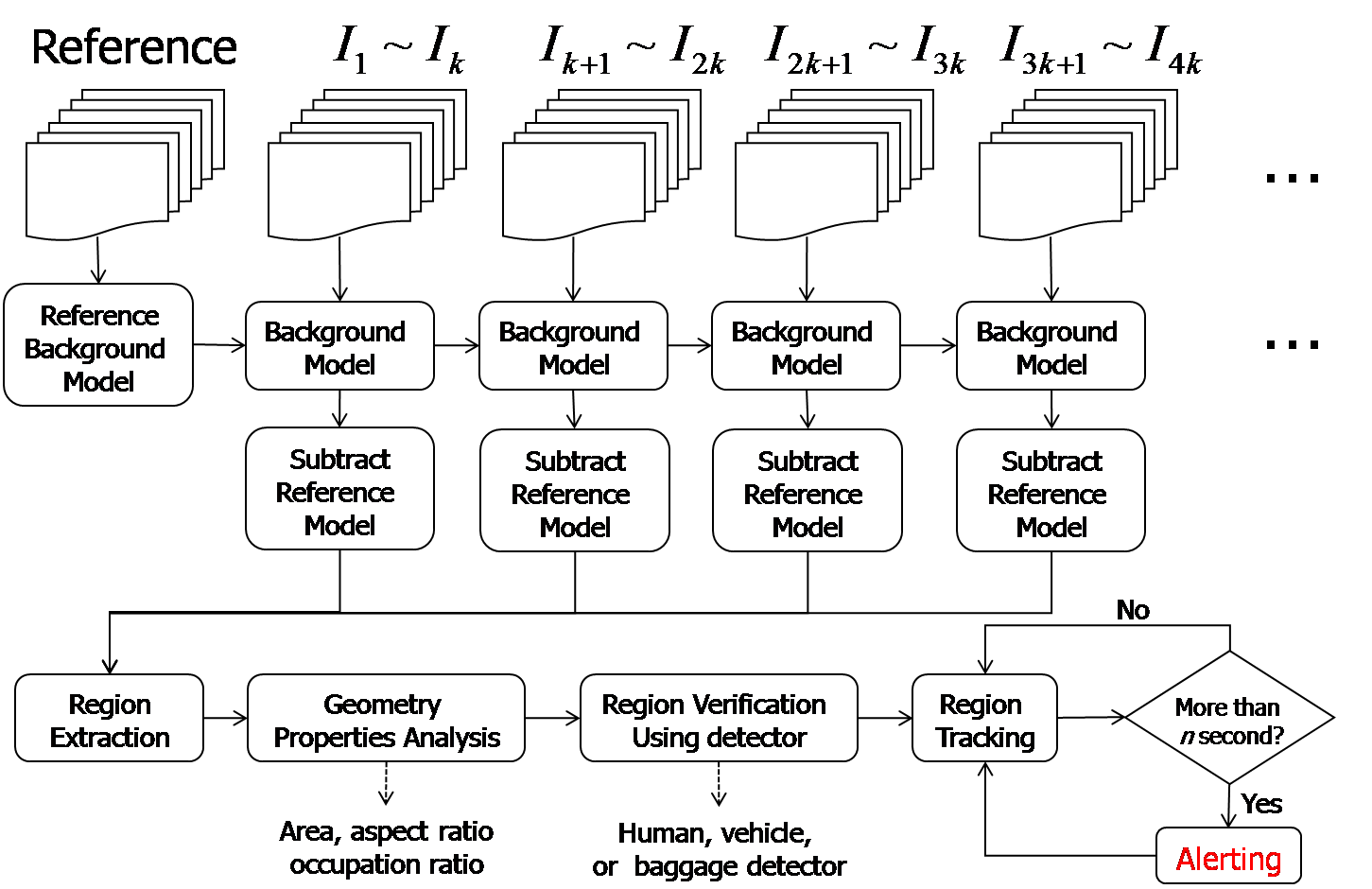
Published on : Wahyono, Alexander Filonenko, and Kang-Hyun Jo, Detecting Abandoned Objects in Crowded Scenes of Surveillance Videos Using Adaptive Dual Background Model, HSI2015, Warsaw, Poland, Jun 25, 2015.
|
Background
In past two decades, developing a system that can navigate vehicle autonomously becomes more interesting problem. The vehicle is equipped by sensors, such as radar, laser, GPS, and camera for sensing the surrounding. Among them, utilization of camera with computer vision technique is the most adopted method for constructing such a system. It is because camera provides a lot of information and is low-cost device rather than other sensors. Traffic road sign, as one of the important information from camera, carries a lot of useful information that are required for navigating.
Methodology
In this work, a system to recognize traffic sign is presented. In a real environment, the traffic signs may have different form and color. Our system consists of four main processes: (1) candidate regions segmentation, (2) shape classification (3) feature extraction, and (4) recognizing. The broadly illustration of our system can be seen at Figure below.
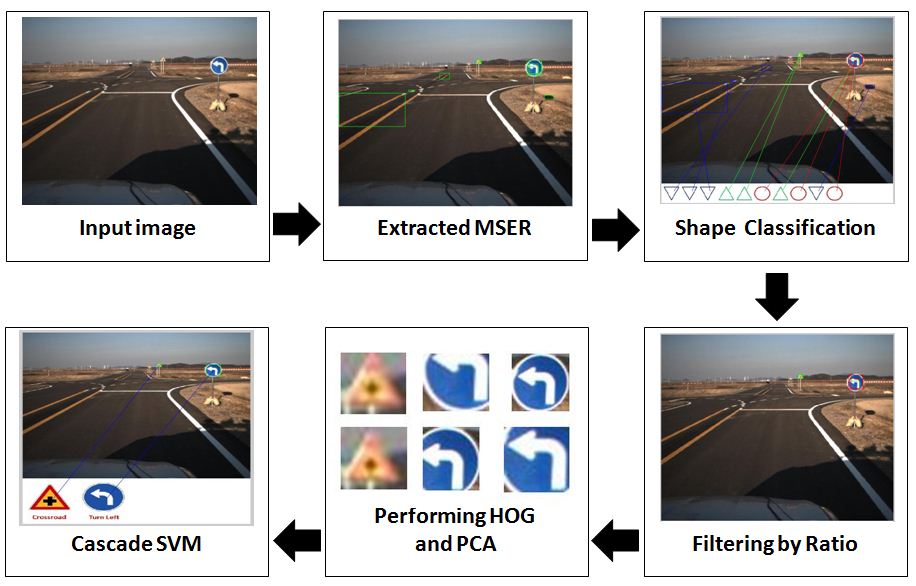
Typical Results
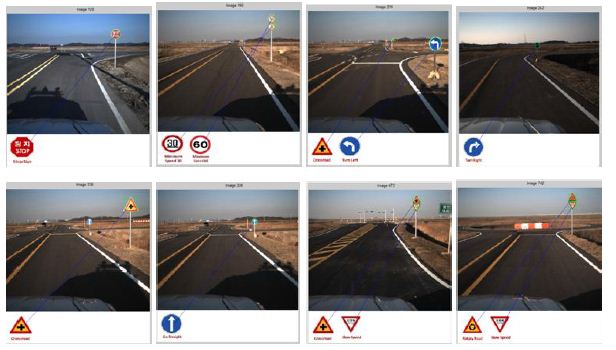
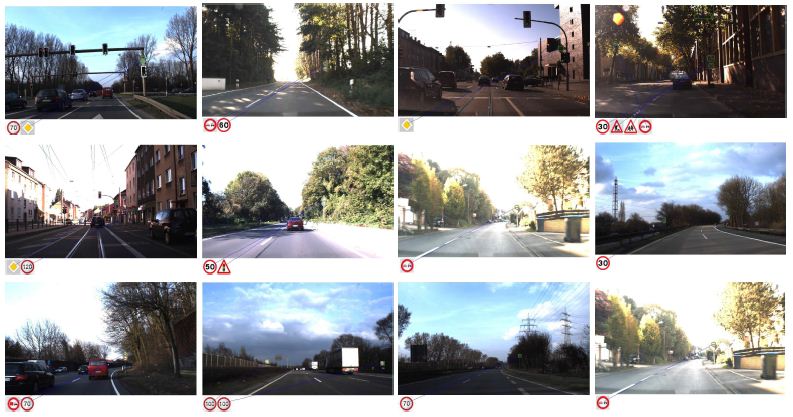
|
|